Vortex on Ice
Using Vortex to accelerate Apache Iceberg queries up to 4x
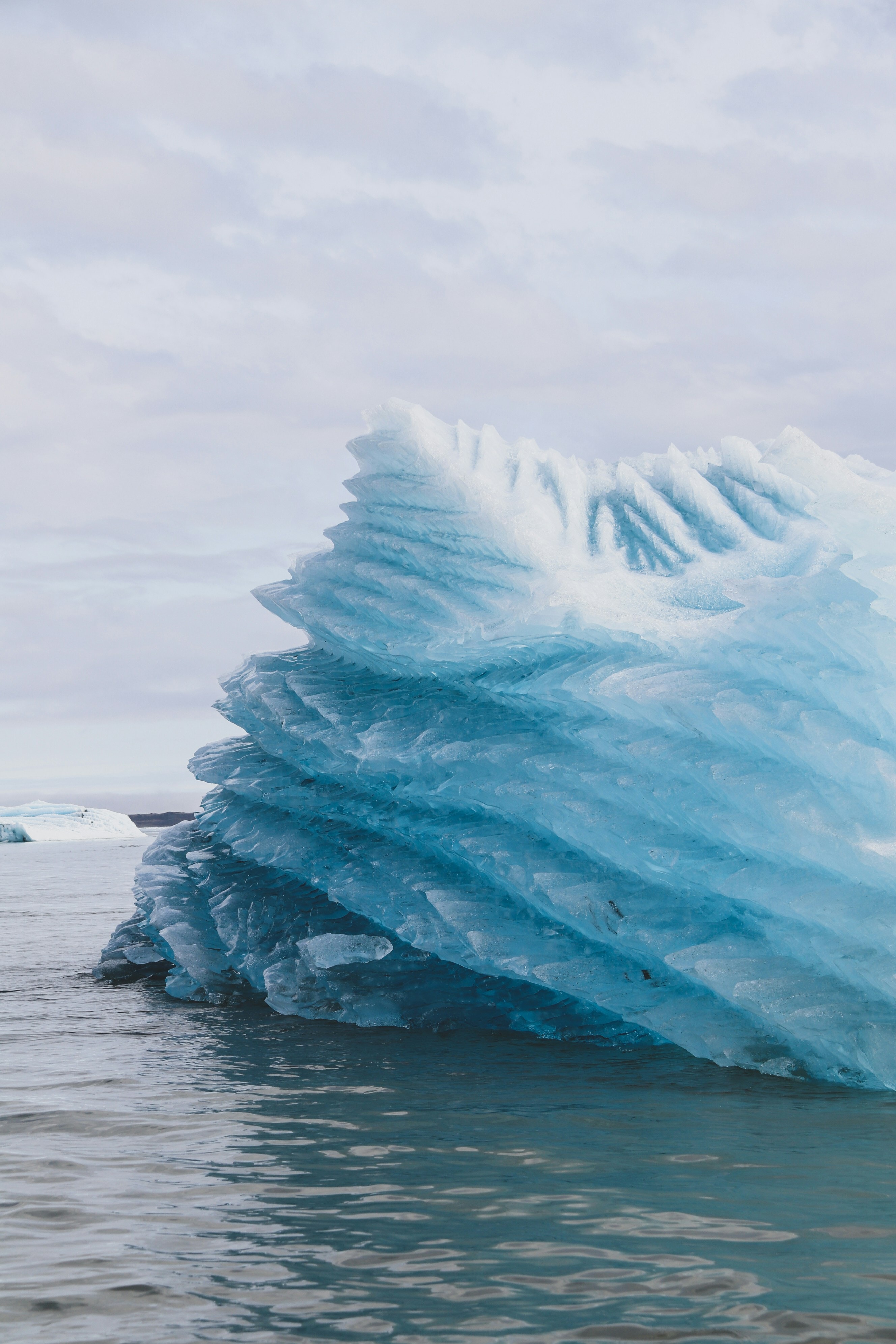
We’ve published new Java bindings for Vortex, and have created an Iceberg fork that uses some in-development APIs to replicate the TPC-H query suite. These results were presented in collaboration with the folks at Microsoft’s Gray Systems Lab at this year’s Iceberg Summit.
If you follow this blog, you’ll be familiar with our open source next-gen file format, Vortex. Vortex was built with an eye toward future data workloads, but it also provides excellent performance on traditional OLAP queries, consistently beating out Parquet in our DataFusion benchmarks for TPC-H and ClickBench.
While we’re very proud of the novel ideas that go into Vortex, we’re most excited about its potential to revolutionize data processing. Across sales calls and conferences, we’ve been struck by the increasing adoption of Apache Iceberg across the industry for both old school analytics and new school AI/ML.
In March, we partnered with the Microsoft Gray Systems Lab to set out to build a tech demo to show Iceberg users how they can accelerate their workloads using Vortex. We presented our results at Iceberg Summit in early April of this year, and we wanted to share more information about how we got to these results, ranging from our approach to building the integration all the way to our experimental setup.
This is a bit longer than our usual posts, one we hope will serve as a detailed record of the dragons slayed on the path to benchmark victory. Feel free to skip ahead to the end if you just want to skim the results. Otherwise, grab your trusty walking stick and get ready to go on an adventure.
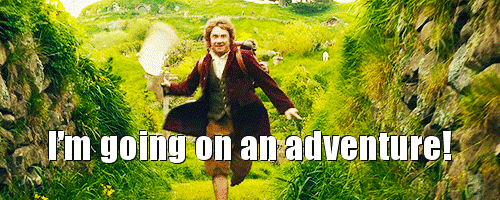
Wither a table format?
The core of every lakehouse system is shared storage, usually in the form of cloud object stores such as S3 or Azure Data Lake Storage. Lakehouses are meant to enable next-generation workloads such as AI training and analytics to live alongside classical BI and reporting workloads. Thus, unlike old-school proprietary data warehouses which silo data behind a SQL-only interface, lakehouse systems want to make it easy to get the data out and into the hands of a variety of downstream consumers. What falls out of this is a new warehouse paradigm where absolutely everything is stored as a collection of files in open formats, the most popular of which being Apache Parquet.
The astute among you may notice that this sounds very similar to a data lake. Data lakes have a perennial problem, which I’ll call “how the hell do I make sense of this giant pile of files??”
As an example, say I have an object storage bucket containing 100,000 observability traces from my production servers. Maybe you’re training an ML model to predict capacity needs for your fleet, so you want to retrieve a dataframe of all events for my hosts in eu-west-1
between 2025-03-27T00:00:00.00Z
and 2025-03-27T01:30:00.00Z
. You also want to setup an hourly job to dump new log events into the lakehouse. And maybe while you’re at it, you want to change the record schema.
Your first instinct might be to treat the bucket as one big ol’ shared drive. Give every dataset a folder, upload new Parquet files into the folder.
And this probably works, for a bit. But you’ll quickly run into all the problems that data lakes failed to solve:
Concurrent access: There is no mechanism for readers and writers to coordinate shared access, leading to data loss and bloated cloud spend
Schema on read: Schema validation is left to the reader, meaning they need to check the schemas of all data files themselves to form the superset of all fields. Schema breakages aren’t found until they’re too late, causing cascading pipeline failures that are difficult to pinpoint and fix
Many small files: Data freshness necessitates frequent appends into the lake, which even for a small cluster of a few dozen nodes you could be dealing with thousands of files every day, making your queries bottle-necked on slow file listing operations
This is where lakehouse systems require a second component: a table format. Table formats provide a management layer over the object store, grouping collections of files into logical tables that offer familiar relational semantics such as schema on write and transaction management. You can think of the table format as the missing link that brings sanity and governance to the lake.
Over the past decade, a slew of competing table standards have cropped up, most of which were spun out of large tech companies as they built tools to tame their massive data lakes. Recently, a clear winner has emerged in Apache Iceberg. Iceberg has gained adoption as the most fully-featured and vendor-neutral option, with teams at Apple, Netflix, Microsoft, Airbnb and many others adopting it for their own internal warehousing needs. Even proprietary warehouses like Snowflake and Google BigQuery have raced to announce integrations, while AWS and Cloudflare have each announced new public APIs that meet the Iceberg specification for their storage customers.
In the dark ages, when Hadoop was still dominant and Spark was but a glitter in Matei’s eye, there was a young table format called Apache Hive. Hive relied on a single relational database that clients could connect to for table discovery and schema information. Tables were partitioned, but partition information needed to be discovered by listing directories in cloud storage buckets, something that is both slow and weakly consistent on most clouds. Additionally, permissions needed to be granted to the Hive Metastore database separately from the underlying file storage, and operating the whole thing was quite a challenge.
In many ways, Iceberg was a response to Hive that prioritized extensibility, operational simplicity, and performance. By reifying the partition information as part of the format, expensive and lossy listing operations could be avoided. Plus, it fits the whole lakehouse vibe of dumping everything into S3 😎🏝️
Iceberg Mechanics
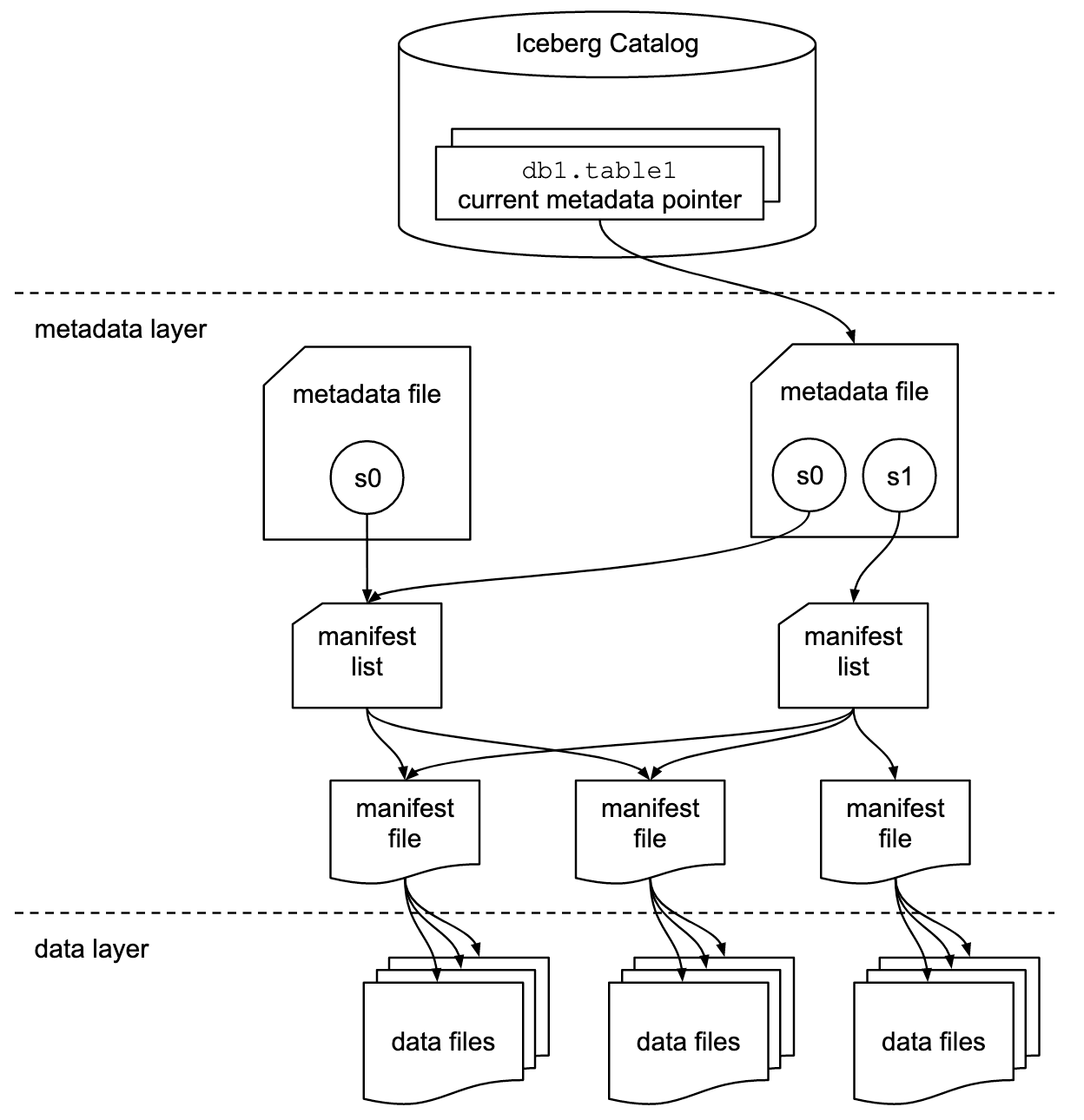
At its core, the Iceberg format is broken down into three layers
Catalog
Metadata files
Data files
Every new write transaction emits several manifest files recording metadata about the files written, such as record counts and some summary statistics. These are further summarized into a manifest list which is part of a metadata file. A client who wishes to read the table starts from a metadata file, traversing the manifest lists and manifests to discover the set of visible files for the query. There can be several available snapshots at a given point in time, allowing you to configure time travel and branch semantics in your own Iceberg deployment.
The catalog sits in front of all metadata and manifest files, providing a central way to create, read, update, and delete Iceberg tables. Iceberg defines a REST Catalog Specification that enables both open and closed players alike to expose their data in an Iceberg-compatible fashion. Providers like Amazon S3 and Cloudflare R2 offer managed catalogs directly on top of storage buckets, but there are also a variety of open providers such as Nessie and Apache Polaris.
The lowest-level component in this architecture are the data files themselves. You know, the files actually containing all of your taxi, citibike, lineitem, and click data. Iceberg allows clients to write data files as Parquet, ORC or Avro, and this decision is currently hard-coded into the core Iceberg codebase. All file IO for supported file types and storage backends is required to live in the main Iceberg codebase as well, posing challenges for us as file format authors that we’ll discuss in the next few sections.
Decoupling the Iceberg
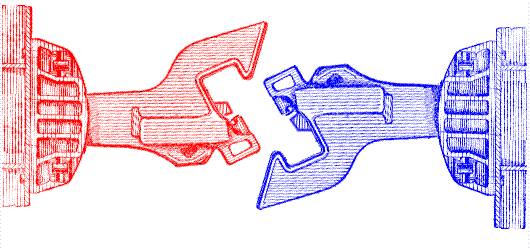
By Dmitry Sutyagin - Own work, CC BY-SA 3.0
Currently, extending the supported formats in Iceberg is a difficult and invasive process, but there’s been work to improve the status quo.
There’s an open pull request from project PMC and Microsoft engineer Péter Váry that seeks to both cleanup the file format logic while at the same time making it easier to extend the set of builtin formats.
To understand why this is necessary, it’s helpful to understand a bit about how the core Iceberg Java implementation works. Fundamentally, Iceberg is a table format that allows file scans over its supported data file formats. These scans can be initiated from one of many supported query engines, and to improve performance they may even want to pushdown predicates and projections to reduce the amount of data read.
When scanning a file, each format needs to specify the in-memory format that batches get read into, for example the ReadSupport interface in Parquet. As an example, say that we wish to initiate a data scan of an Iceberg table consisting of ORC files, each with fields (X Y Z)
, and we’d like to execute the query SELECT Y, Z FROM table WHERE X = 3
using Apache Spark SQL. The sequence of steps that Iceberg internally executes looks something like
Convert the Spark filter expression
X = 3
into an equivalent Iceberg expression.Traverse all manifest files reachable from the current snapshot to identify all ORC data files visible for the query. If your files are partitioned, this also requires converting the Spark filter expression into an Iceberg filter expression that can be used to prune partitions.
Generate Spark tasks to scan each matched ORC file.
Convert the Iceberg filter expression into an ORC
SearchArgument
and pushdown the projectionY,Z
. Wrap the resulting stream ofVectorizedRowBatch
into SparkColumnarBatch
for vectorized processing.Spark will re-apply the
X = 3
predicate after the scan in case the pushed filter result was inexact.
Depiction of the steps for Iceberg's predicate and projection pushdown when using Spark.
The ORC nomenclature of SearchArgument
is derived from the relational algebra term sargable , which in traditional relational DBMS jargon just means a query column that has an available index which accelerates filtering. In the case of columnar formats like ORC and Vortex, explicit index structures are dispensed with in favor of Zone Maps, an approach we cover extensively in another post.
Every step in this process is specialized to a specific pair of file format and execution engine.
The new interface promulgated by the PR seeks to streamline the process by creating separate generic and engine-specific implementations for each file format. We encourage interested readers to look directly at the PR discussion for more details, as well as the conversation on the mailing list.
We developed our own fork of Iceberg based on the PR that served as a basis for all of our testing. We have focused on building a competitive vectorized Spark reader for Vortex files that we put head-to-head with Iceberg’s vectorized Parquet reader for Spark.
All the currently supported file formats have reference Java implementations, Parquet, Avro and ORC and quite well-situated. There is just one problem: Vortex is written in Rust! What should we do to implement support?
One option is to build fresh Java implementations of all of the Vortex encodings, similar to how there are dozens of Parquet implementations out there. However, one of the core project goals with Vortex was to enable format evolvability , something Parquet has struggled with in part due to the lack of a single reference implementation. As new features such as the Page Index or Bloom Filters were added over time, different implementations pickup support for them on different schedules, meaning it can take years to get the entire community to coordinate on the rollout.
Another big reason to avoid rewriting the encodings in Java are to preserve predictable performance. We go to great lengths in Vortex to optimize the performance of our lightweight encodings. Care is taken to reduce unnecessary allocations and code is profiled to make sure that it auto-vectorizes well across common platforms. This level of control is only possible in a native language like Rust. Java and the JVM’s builtin JIT provide a lot of power to perform optimizations like this, but it quickly becomes difficult to wield and reason about.
Thankfully, there is another option, one that allows us to bind our existing library to Java directly!
Vortex and a cup of Joe ☕️
Java was first released in the 1990s at the height of Moore’s Law and the coincident Cambrian Explosion of CPU architectures. It had the lofty goal of freeing developers from the tedium of rebuilding their applications for every available platform. It was trying to achieve the dream of being a “write-once, run-anywhere” language, based on an advanced JIT virtual machine runtime that could be run on any machine from a desktop computer all the way down to a microcontroller. Over time it became used for more than just GUIs, making its way into network services and databases, and calling into native code became a necessity. In release 1.3 they announced the Java Native Interface specification, which outlines how to bind class and instance methods to native code.
This allowed you to write a Java classes like this
package com.example.geo;
public class GeoLocation {
public static native String formatLocation(
long latitude,
long longitude);
}
See the native
keyword on the method declaration? That tells the compiler that the method is implemented by a shared library which will be loaded at runtime, by a function with the following name and signature
#include <jni.h>
JNIEXPORT jstring JNICALL Java_com_example_geo_GeoLocation_formatLocation
(JNIEnv *, jclass, jlong, jlong);
The C ABI is the secret sauce here, providing a lingua franca for calling functions across languages that use the C function-calling convention.
You’ll probably notice, JNI brings in a lot of cognitive overhead, and forces you to write JNI wrappers, even if you already have some C ABI-compatible functions lying around.
There are many excellent projects such as Java Native Access and javacpp that attempt to make it easier to work with native code that have found a lot of success, and the JVM Language Team has been working to incorporate Project Panama, which landed native code calling as a preview API in Java 19.
Our first instinct was to use Project Panama and build on their many years of work in this space, but unfortunately the Iceberg project requires building for Java 11 targets as a lowest common denominator, and Spark only supports Java up to version 17.
javacpp
is very C++ focused, and requires generating code from header files, so we decided not to investigate it further. Our next attempt was to use Java Native Access, which was the basis of our first native bridge from Vortex to Java. However, this initial prototype saw really poor performance in SQL benchmarks. In our own microbenchmark testing, we found JNI to be up to 3x faster than JNA for even simple operations like String copying. This led us to migrate to direct usage of the JNI, which is the current state of affairs.
There is a jni
Rust crate that wraps the JNI complexity a bit for us, allowing you to write Rust functions similar to the C++ header we saw above:
use jni::objects::{JClass, JString};
use jni::sys::{jlong, jstring};
use jni::JNIEnv;
// `no_mangle` ensures that the compiler leaves the symbols name intact.
#[unsafe(no_mangle)]
pub extern "system" fn Java_com_example_geo_GeoLocator_getAddress(
env: JNIEnv,
_class: JClass,
lat: jlong,
lon: jlong,
) -> jstring {
let rust_string = format!("({lat}, {lon})", lat, lon);
let output = env
.new_string(rust_string)
.expect("Couldn't create java string");
output.into_raw()
}
Rust library crates generally build to .rlib
files, which can be consumed by other Rust crates. But you can also enable building shared libraries by adding a configuration to your Cargo.toml
[lib]
crate-type = ["cdylib"]
Alright, so we have established that we want to reuse our Rust implementation of the Vortex format, and expose it to Java code via JNI. This leads to the next problem, which is one of API design. As we sketched out earlier in the post, we need to expose an interface to Iceberg that allows us to
Open a handle to a Vortex file, possibly with some parameters (e.g. the Azure credentials)
Create a new file scan with pushed projection and predicates
Export the scan result as a stream of Arrow batches to hand back to the parent Spark operator
Let’s start from the mindset of designing a Java API for this component. We might define some NativeFile
class with a constructor that takes the connection parameters, and provides a method to build a new scan. Perhaps something like this:
public class NativeFile {
private final URL url;
public NativeFile(URL url) {
this.url = url;
}
public void open() throws IOException {
// Open a connection to Azure Data Lake Store using our URL
// and properties.
}
// Create a new file scan, pushing the given Iceberg
// predicate down to Vortex.
public Scan newScanner(Expression predicate) {
// ?
}
}
// A Scan is just an iterator that yields Arrow columnar batches
public class Scan implements Iterator<ArrowColumnVector> {
public Scan(ArrayIterator iterator) {
this.iterator = iterator
}
@Override
public void hasNext() {
return iterator.hasNext();
}
@Override
public ArrowColumnVector next() {
return iterator.next();
}
}
Seems simple enough! Let’s try and implement the open
method on NativeFile
. In Rust, we have a VortexFile, so we just want to build one of those, save it as an instance variable, and…
Wait a minute. We can’t save a Rust struct in Java! What would its type be?
We need to build Rust objects, and pass a handle to them back over the FFI to Java. The way we pass a handle from Rust to another language is to pass a pointer to something with a ’static
lifetime. The easiest way to create an object with a 'static
lifetime is to heap-allocate it, which in Rust means putting it inside of a Box<T>
. A Box
is just a wrapper around a memory allocation, and in Java a pointer is just another long
(or a jlong
in jni
crate parlance). We can use Box::into_raw
to unwrap our allocation into its raw pointer form, and pass the address as the return value of the Java method.
So here’s our Java code for opening a file:
public final class NativeFileMethods {
// This class only defines static methods, and should never
// be instantiated by a call to `new`. Making its constructor
// private prevents it from accidentally being used in ways
// we didn't intend.
private NativeFileMethods() {}
/**
* Open a file using the native library with the provided URI
* and options.
*
* @param uri The URI of the file to open.
* e.g. "file://path/to/file".
* @param options A map of options to provide for opening
* the file.
* @return A native pointer to the opened file.
* This will be 0 if the open call failed.
*/
public static native long open(
String uri,
Map<String, String> options
);
}
And here’s our Rust code
/// Native implementation for dev.vortex.jni.NativeFileMethods.open()
#[unsafe(no_mangle)]
pub extern "system" fn Java_dev_vortex_jni_NativeFileMethods_open(
mut env: JNIEnv,
_class: JClass,
uri: JString,
options: JObject,
) -> jlong {
// try_or_throw is a helper that runs a closure that returns a Result,
// and if it returns an error it throws it as a RuntimeExcpetion
// back to the JVM.
try_or_throw(&mut env, |env| {
// Copy the string from the Java heap to Rust heap
let file_path: String = env.get_string(&uri)?.into();
let Ok(url) = Url::parse(&file_path) else {
throw_runtime!("Invalid URL: {file_path}");
};
// Some helper to make the ObjectStore for the URL
let store = make_object_store(&url)?;
// block_on is a helper to execute a future in a blocking fashion
// on the current thread.
let open_file = block_on(
"VortexOpenOptions.open()",
VortexOpenOptions::file().open_object_store(
&store,
url.path()
),
)?;
// Put the VortexFile onto the native heap, and return
// the raw pointer to the caller as a Java long.
Ok(Box::new(open_file).into_raw())
})
}
But wait a minute: Box
depends on its Drop
impl to cleanup the memory it allocated, so by turning it into a raw pointer and consuming the Box
, we have leaked this memory! Luckily, Box
provides an unsafe from_raw
function that can turn a raw pointer returned from an earlier call to Box::into_raw
back into a Box<T>
, which can then get dropped, freeing the native memory.
This is a simple matter of adding a close
method in Java:
/**
* Close the file associated with the given pointer.
*
* @param pointer The native pointer to a file.
*/
public static native void close(long pointer);
And Rust:
#[unsafe(no_mangle)]
pub extern "system" fn Java_dev_vortex_jni_NativeFileMethods_close(
_env: JNIEnv,
_class: JClass,
pointer: jlong,
) {
// SAFETY: the pointer MUST be a value returned from a previous
// call to NativeFileMethods.open() and be non-zero.
drop(unsafe { Box::from_raw(pointer as *mut VortexFile) });
}
This works, but in the process, we’ve effectively turned this Java class into a thin layer over malloc
/ free
. This is to some degree unavoidable: the Java class that owns the pointer now needs to manually manage its lifetime. However, history has shown that manual memory management is hard and when done poorly, can lead to all sorts of bugs.
The NativeFileMethods
class is a footgun, ideally we’d hide it so that it’s not generally accessible, and instead provide some porcelain interface that makes it clear how to use correctly. Ideally such an interface would also prevent memory-unsafe operations, into throwing Java exceptions when a memory corruption would’ve otherwise ocurred.
Here’s what we can do: we create a new JNIFile
wrapper class, that is much closer to the original VortexFile
Java class we wrote at the beginning of this section. It has a constructor that receives arguments to open a file, and saves the native pointer. Crucially, rather than saving it as a long
, it uses OptionalLong
. Something like this
public final class JNIFile {
private OptionalLong pointer;
public JNIFile(String uri) {
this.pointer = OptionalLong.of(NativeFileMethods.open(uri));
}
}
Now, there’s a neat trick this enables: we can make JNIFile
implement AutoCloseable
, and tie the lifetime of the pointee to the lifetime of the object from the time is it constructed up until it is closed. Our implementation of the close()
method will free the native memory, and empty the OptionalLong
. The lifecycle now looks like
Create a
new JNIFile
, also creating native resources like memory allocations, network socketsUse it to read data
Call
JNIFile.close()
, freeing the native resources and marking the pointer as anOptionalLong.empty()
Any attempt to use the pointer will go through OptionalLong.getAsLong
, which will return a NullPointerException
if it’s empty. We’ve turned operations that would have resulted in either a double-free or use-after free into a Java exception, allowing us to preserve some semblance of memory safety even outside of Rust.
Let’s imagine for example that there is another long NativeFileMethods.rowCount(long pointer)
that returns the number of rows in the file. By forcing an unwrap of our pointer, we’ll prevent runtime usage of the JNIFile
after it’s been closed:
public final class JNIFile implements AutoCloseable {
private OptionalLong pointer;
public JNIFile(String uri) {
this.pointer = OptionalLong.of(NativeFileMethods.open(uri));
}
public long getRowCount() {
// pointer.getAsLong() will throw NoSuchElementException if
// this method is called after close was called.
return NativeFileMethods.rowCount(pointer.getAsLong());
}
@Override
public void close() {
// This will throw an exception if close has
// already been called.
NativeFileMethods.close(pointer.getAsLong());
// Clear the pointer handle, preventing
// access to the freed memory.
pointer = OptionalLong.empty();
}
}
We found this to be a fairly productive pattern to use when implementing code that crosses the Rust/Java boundary, and was the basis for our Iceberg integration.
Benchmarking the integration
Our benchmarking setup was done on Azure using an E8ads
Virtual Machine with 8 cores and 64GB of memory, the same VM profile used for the LST-Bench evaluations. We ran a single-node Spark configured with
Statically allocated 31GB of JVM driver memory
8 driver cores
We used the TPC-H dataset at scale factor 100. Data was stored in an Azure Data Lake StorageV2 container in the same region as the VM.
We took the DuckDB TPC-H dataset and exported it to Parquet, with the following settings:
Maximum size 1GB
Row group size 512MiB
Page index enabled
For every Parquet file, we generated an equivalent Vortex file using default compressor settings using the vx
CLI tool.
The JAR was built of our test harness code and uploaded to the cluster via spark-submit
, which warms the SparkContext before running the full test suite for both Vortex and Parquet.
The first run, we were mainly neck-and-neck with Parquet, but for large joins such as Q9 we were up to 2x slower. What gives?
We pulled up our profiler while the query was executing and noticed that all of our task stack traces looked something like this:
java.base@17.0.14/sun.nio.ch.FileDispatcherImpl.write0(Native Method)
java.base@17.0.14/sun.nio.ch.FileDispatcherImpl.write(FileDispatcherImpl.java:62)
java.base@17.0.14/sun.nio.ch.IOUtil.writeFromNativeBuffer(IOUtil.java:132)
java.base@17.0.14/sun.nio.ch.IOUtil.write(IOUtil.java:76)
java.base@17.0.14/sun.nio.ch.IOUtil.write(IOUtil.java:67)
java.base@17.0.14/sun.nio.ch.FileChannelImpl.write(FileChannelImpl.java:288) => holding Monitor(java.lang.Object@439399089)
java.base@17.0.14/sun.nio.ch.FileChannelImpl.transferToTrustedChannel(FileChannelImpl.java:605)
java.base@17.0.14/sun.nio.ch.FileChannelImpl.transferTo(FileChannelImpl.java:699)
app//org.apache.spark.util.Utils$.copyFileStreamNIO(Utils.scala:346)
app//org.apache.spark.util.Utils.copyFileStreamNIO(Utils.scala)
app//org.apache.spark.shuffle.sort.UnsafeShuffleWriter.mergeSpillsWithTransferTo(UnsafeShuffleWriter.java:465)
app//org.apache.spark.shuffle.sort.UnsafeShuffleWriter.mergeSpillsUsingStandardWriter(UnsafeShuffleWriter.java:321)
app//org.apache.spark.shuffle.sort.UnsafeShuffleWriter.mergeSpills(UnsafeShuffleWriter.java:288)
app//org.apache.spark.shuffle.sort.UnsafeShuffleWriter.closeAndWriteOutput(UnsafeShuffleWriter.java:224)
app//org.apache.spark.shuffle.sort.UnsafeShuffleWriter.write(UnsafeShuffleWriter.java:182)
app//org.apache.spark.shuffle.ShuffleWriteProcessor.write(ShuffleWriteProcessor.scala:59)
app//org.apache.spark.scheduler.ShuffleMapTask.runTask(ShuffleMapTask.scala:104)
app//org.apache.spark.scheduler.ShuffleMapTask.runTask(ShuffleMapTask.scala:54)
app//org.apache.spark.TaskContext.runTaskWithListeners(TaskContext.scala:166)
app//org.apache.spark.scheduler.Task.run(Task.scala:141)
app//org.apache.spark.executor.Executor$TaskRunner.$anonfun$run$4(Executor.scala:620)
app//org.apache.spark.executor.Executor$TaskRunner$$Lambda$2794/0x000072c48cea47e0.apply(Unknown Source)
app//org.apache.spark.util.SparkErrorUtils.tryWithSafeFinally(SparkErrorUtils.scala:64)
app//org.apache.spark.util.SparkErrorUtils.tryWithSafeFinally$(SparkErrorUtils.scala:61)
app//org.apache.spark.util.Utils$.tryWithSafeFinally(Utils.scala:94)
app//org.apache.spark.executor.Executor$TaskRunner.run(Executor.scala:623)
java.base@17.0.14/java.util.concurrent.ThreadPoolExecutor.runWorker(ThreadPoolExecutor.java:1136)
java.base@17.0.14/java.util.concurrent.ThreadPoolExecutor$Worker.run(ThreadPoolExecutor.java:635)
java.base@17.0.14/java.lang.Thread.run(Thread.java:840)
It’s not even in our code at all! So what’s happening?
It all goes back to Iceberg’s idea of file “splittability”. When Iceberg plans to read a bunch of files to execute the query, it first checks to see if the files are in a splittable format. If they’re splittable, they divide it up into roughly evenly sized byte ranges, and create one Spark task to read the data from each byte range. This works for Parquet and other pure PAX formats because a set of column chunks forms a contiguous region in the file. In Vortex, however, that’s not the case. We achieve parallelism via our flexible Layout mechanism, which does not align horizontal splits as contiguous byte ranges, so we were reporting Vortex as unsplittable. This forced Iceberg to plan a single task for each Vortex file, each of which contained tens of millions of tuples. Because our tuples are so large, this meant that the shuffle data needed to be spilled to several files, which were then merged at the end of the stage. This is an extremely slow operation, one that is easily solved by planning smaller map tasks.
To address this, we added a new concept of “row-splittability”, that allows us to report desired row-splits rather than byte-range splits in Iceberg metadata files. This enabled Spark to generate the same level of parallelism for Vortex reads as it did for Parquet reads, leveling the playing field.
Once we’d implemented our new row-splitting additions to the planner, we ran our benchmarks again, and found much more enticing results!
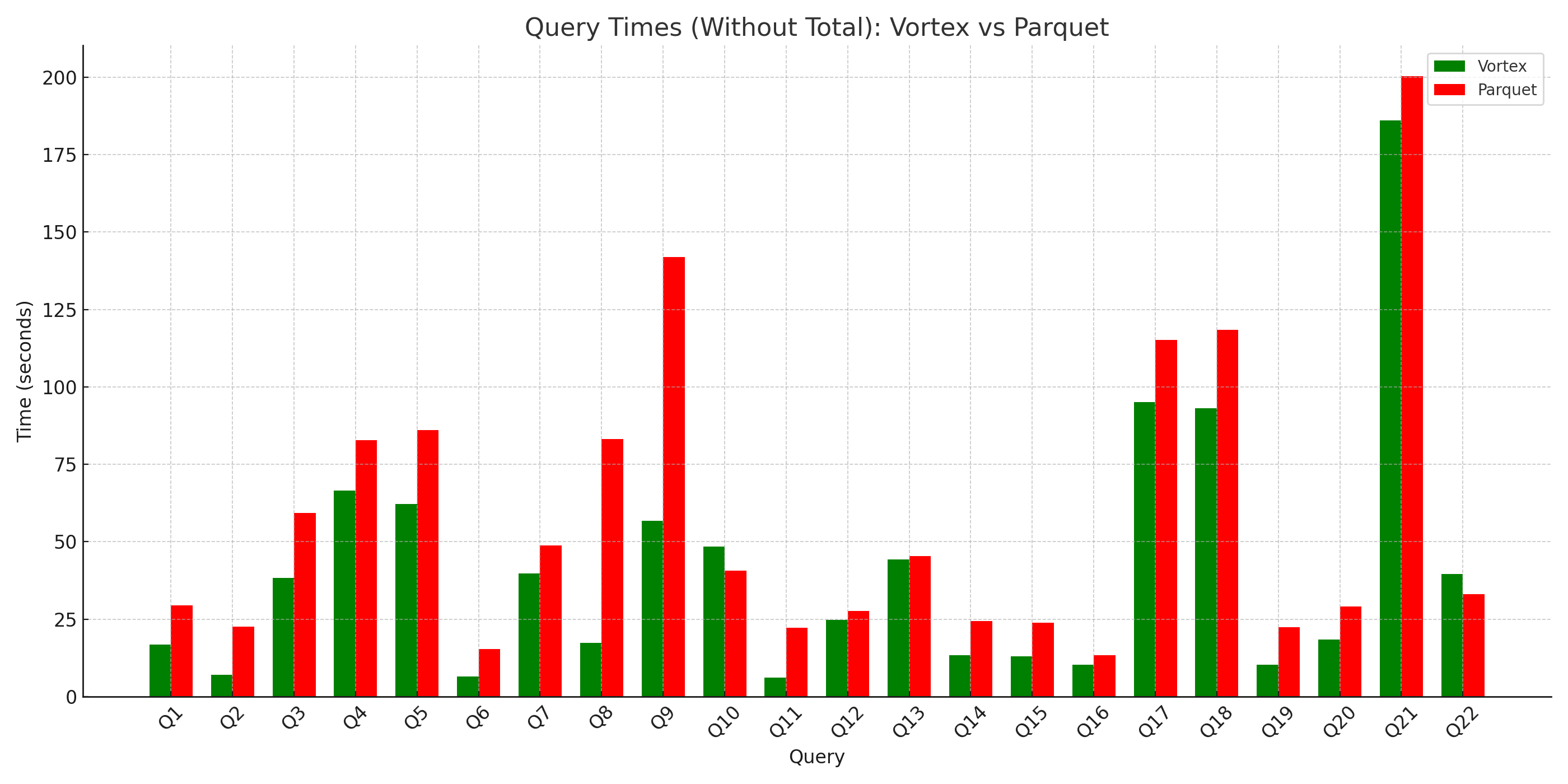
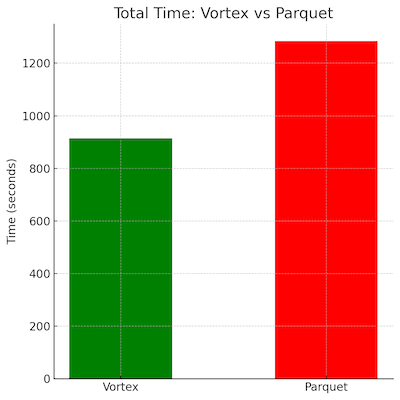
We were able to achieve a nearly 30% speedup on the whole suite, with improvements of 2-4x for some of the slowest queries. Decoding into Arrow is much faster with Vortex than Parquet due to its exclusive use of lightweight compression methods and its Arrow-compatible in-memory format. We also benefit from improved pruning due to our granular zone maps, allowing us to not only skip reading whole chunks, but actually selectively decoding extents within a chunk.
The road goes ever on and on
We wanted to give a huge thanks to Péter Váry, Ashvin Agrawal, and Carlo Curino from Microsoft Gray Systems Lab for their continued work on making Iceberg more pluggable for the broader ecosystem, as well as for sponsoring our Azure test cluster and answering our questions about Apache Iceberg along the way.
Getting to the demonstration was an exciting milestone, but there’s still so much more to do, including
Continuing to collaborate with the community to standardize the
FileFormat
APIImplementing support for Deletion Vector pushdown into Vortex, allowing lightning-fast merge-on-read with scattered position deletes (in-progress PR)
Support for Iceberg-level encryption
If you’re excited about giving Vortex a shot in your Iceberg warehouse, please reach out! Until then, stay frosty folks 🧊
Recommended posts
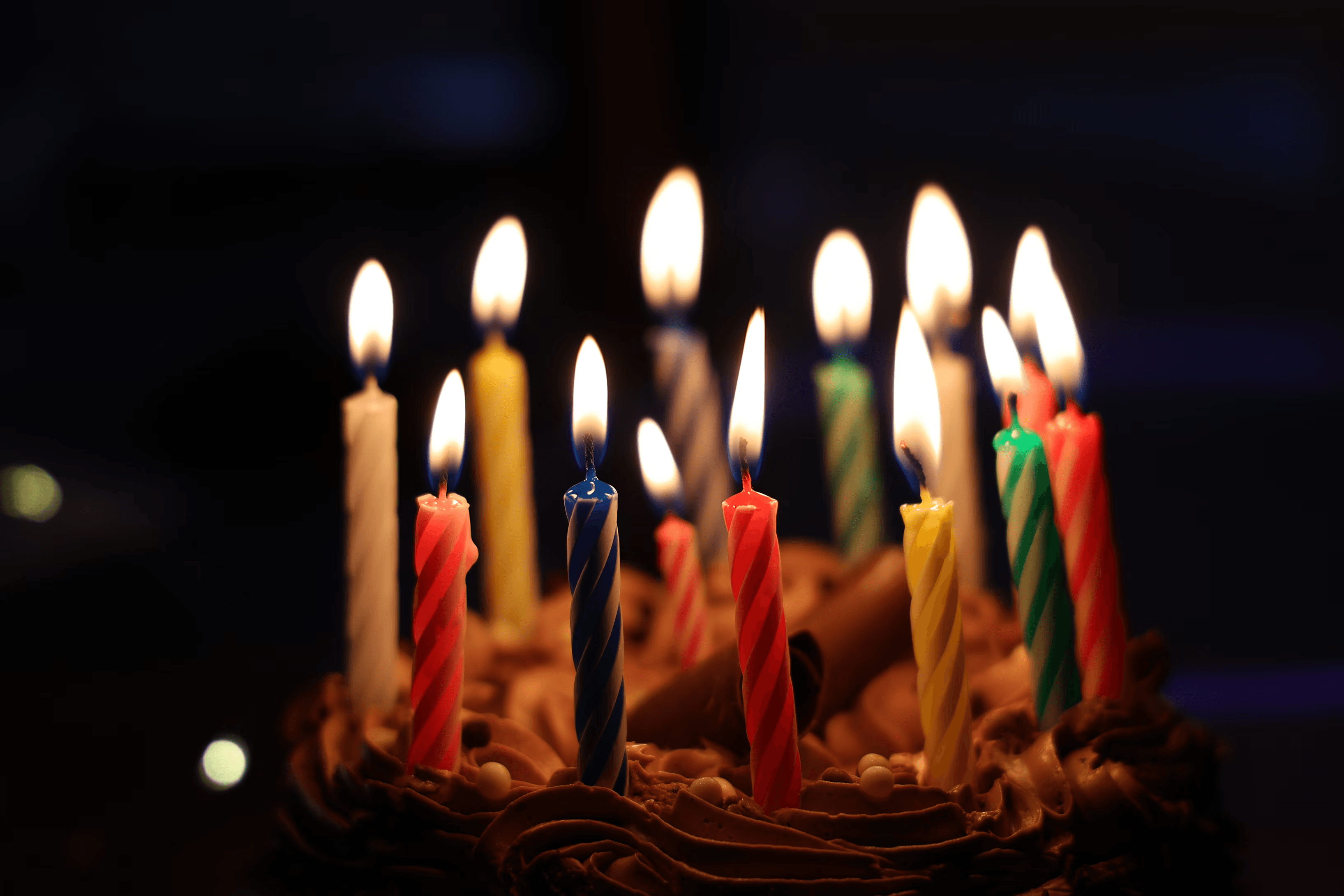
Towards Vortex 1.0
Preparing to launch Vortex 1.0 and the 2025.05 Edition
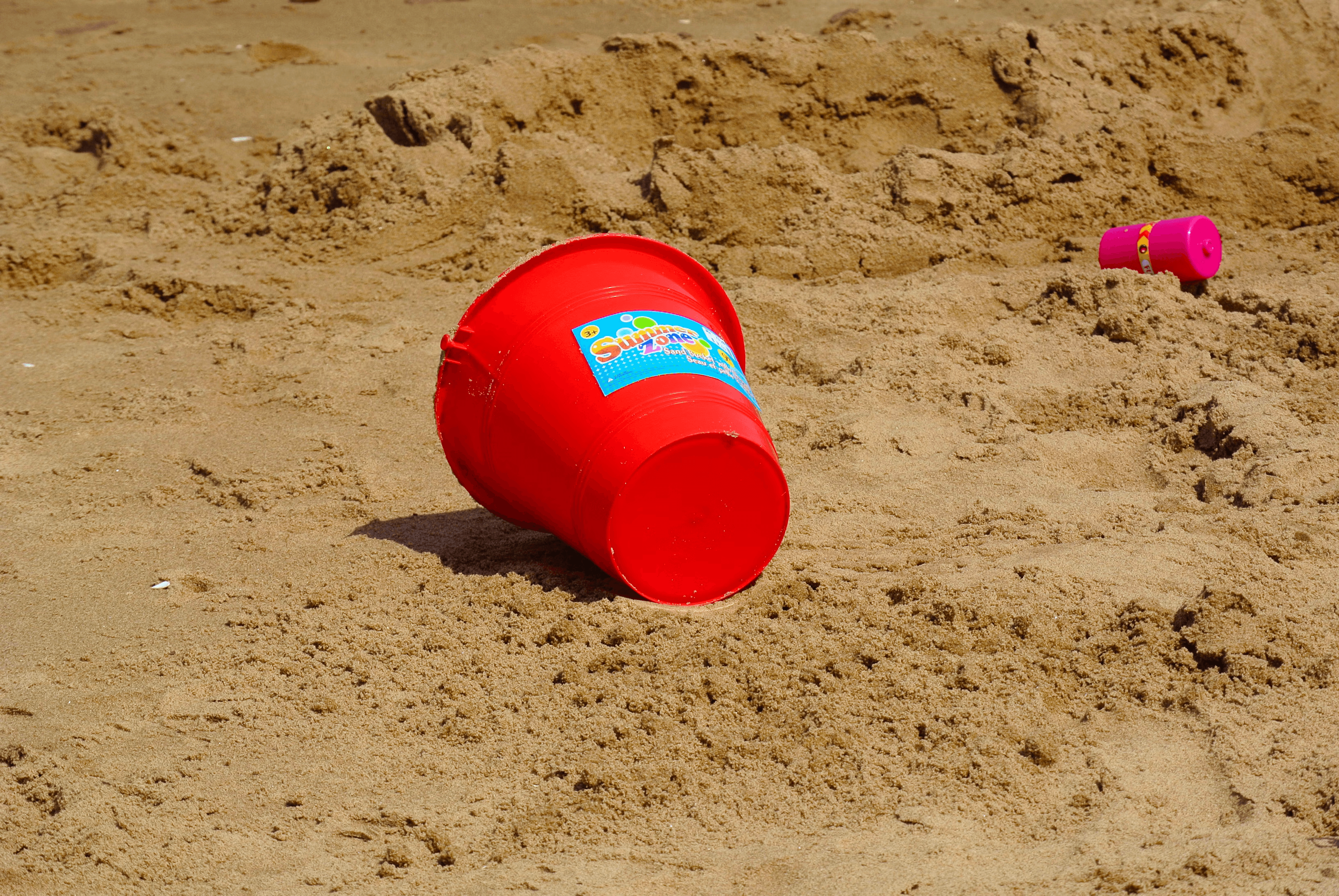
So you want to use Object Storage
Tips and lessons learned from building systems directly against object stores
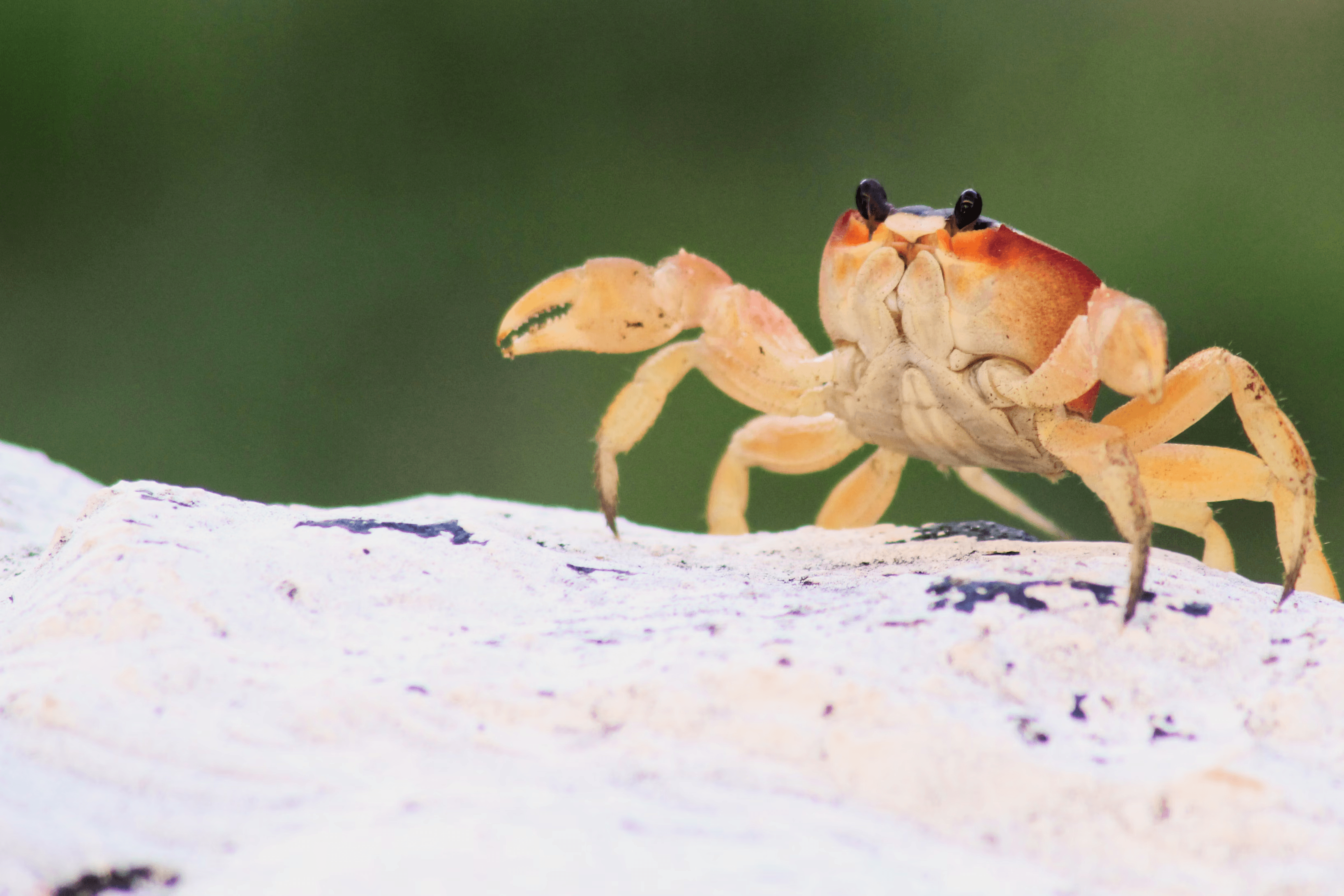
ALP Rust is faster than C++
How well-defined casting semantics in Rust allow for 20-50% faster ALP compression